Crowdsourcing Knowledge-Intensive Tasks In Cultural Heritage
Poster at WebSci’14, June 23–26, 2014, Bloomington, IN, USA.
Corresponding author: Jasper Oosterman, j.e.g.oosterman@tudelft.nl
Abstract
Large datasets such as Cultural Heritage collections require detailed annotations when digitized and made available online. Annotating different aspects of such collections requires a variety of knowledge and expertise which is not always possessed by the collection curators. Artwork annotation is an example of a knowledge intensive image annotation task, i.e. a task that demands annotators to have domain-specific knowledge in order to be successfully completed.
This paper describes the results of a study aimed at investigating the applicability of crowdsourcing techniques to knowledge intensive image annotation tasks. We observed a clear relationship between the annotation difficulty of an image, in terms of number of items to identify and annotate, and the performance of the recruited workers.
Click here for the full (2 pages) poster paper.
Extended Experimental Setup in addition to the paper
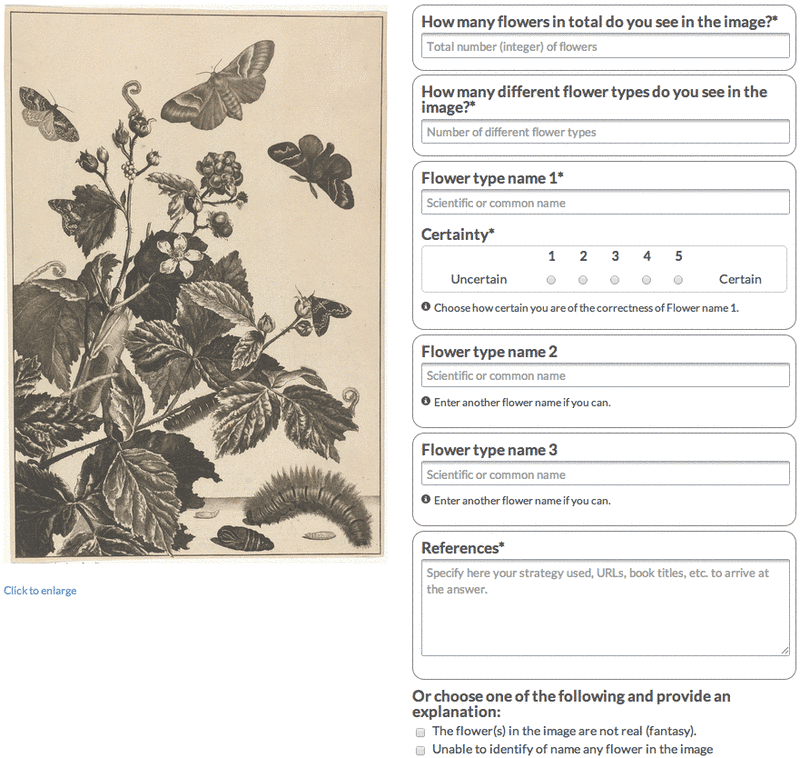
Fig 1. User interface for annotators
The experiment involved anonymous annotators drawn from the CrowdFlower platform, which recruits workers worldwide. Based on our previous experience in similar tasks, and after several pilot experiments, we set the compensation per task (the annotation of five prints) to 18 cents. We also selected the Level 1 Contributors option of CrowdFlower and excluded the following countries from participation: Colombia, Estonia, India, Latvia, Romania, Vietnam. Level 1 contributors are high performance contributors who account for 60% of monthly judgments and maintain a high level of accuracy across a basket of CrowdFlower jobs.
Figure 1 depicts the user interface of the testbed designed and implemented for the experiment. The print to annotate is presented on the left hand side. The worker can open the image at full screen by clicking on it. The right hand side lists the annotation fields. First, workers are requested to indicate the number of flower instances and the number of flower types they were able to identify. As each print contains at least one flower, in order to complete the task an annotator must provide at least one flower type name. Three text fields are available to provide at least one and up to three names of the type of flowers in the print. No auto-suggestion or auto-correction functionalities are provided. The worker is requested to enter the botanical name or the common name of the depicted flower(s).
When a label is provided, a 5-point Likert scale field allows workers to self-assess their confidence about the annotation (from 1 for uncertain to 5 for certain). Workers are instructed to specify in the "References" text box the flower recognition method (e.g. Web search strategy, URLs, books titles, etc.) adopted to produce their answer. To account for flowers that cannot be identified or named by the workers, they can specify if the prints contain flowers of imaginative nature (fantasy), or when they are unable to name any identified flower (unable). In both cases, an additional text box is prompted to collect comments about the reasons for their judgment.
Each annotation task is introduced by a description page, listing instructions about the requested input, an explanation of the user interface, and examples of target annotations. It also includes a definition of when to count a flower and when not. Each annotator is requested to perform first a test task, a necessary pre-condition to participate in the rest of the experiment. The test task consisted of the annotation of five prints, using the user interface in Figure 1. The prints were sampled from the set of prints belonging to the easy-easy class. For each print, we compared the number of flowers and the number of flower types provided by the annotator to our ground-truth. Workers who failed more than one test question were rejected, and thus not allowed to perform any annotation tasks. Successful workers could continue to execute one or more tasks. To avoid learning bias, prints in the test task were not offered for annotation in the subsequent tasks. Each annotation task contained five prints; in each task, one easy-easy print has been used as an additional quality control mechanism by matching the specified number of flowers and number of flower types annotations against our ground-truth. Prints were shown to workers in random order. Each worker could annotate every remaining print in the set, but only once. We designed the task such that each image could be annotated by at most 10 annotators. The maximum task duration was set to 15 minutes. If, across all executed tasks, the overall accuracy on easy-easy prints of a worker dropped below 60%, then the annotation of such workers was discarded.
Dataset
The dataset consists of annotations created by 5 manually contacted experts and crowd workers contacted via CrowdFlower. This dataset is made available supporting our submission for the Web Science 2014 conference: Crowdsourcing Knowledge-Intensive Tasks in Cultural Heritage.
Click here for the archive of the dataset.
Files in dataset
- dataset_complete.csv
- The collection of 82 prints used in the experiment
- expert_labels.csv
- The collected labels from the experts
- f7383708_with_untrusted.csv
- The collected data from crowd workers unfiltered by CrowdFlower
- workset383708.csv
- The set of users who interacted with the tasks
- flowercrowds.R
- The R script used to process the data
Poster
The poster will appear here after the conference.